Publicité
“Winning against cancer is one of the holy grails of medical research”
Par
Partager cet article
“Winning against cancer is one of the holy grails of medical research”
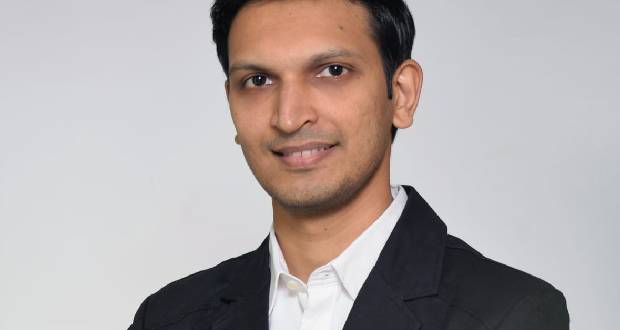
A major breakthrough in cancer diagnosis research using Artificial Intelligence makes the headlines of several international publications. At the heart of it is a compatriot originating from Grande Retraite, a small village in the east of Mauritius. We approached him to tell us more about how this research is going to make an impact in the fight against cancer and how he made his way from a village many had never heard of to one of the most prestigious research centres. A success story you don’t want to miss.
You made the headlines about a breakthrough in research into cancer diagnosis using Artificial Intelligence. Tell us a bit about how AI is used in this technology.
AI refers to different types of systems which can simulate certain aspects of human intelligence and take certain decisions without being explicitly told how to take them. The flavour of AI we have used in this project is known as Machine Learning (ML). It is a subgroup consisting of programmes which can learn by analysing a large number of examples. In a way, it examines decisions taken by humans and learns its own rules for performing similar tasks in the future.
So how did you use AI here?
We have employed AI to perform two main tasks here. Firstly, to identify every single cell present in large images of crowded populations of cells. And secondly, to determine the disease status of each cell based on the acidity patterns within the cell.
Why did you have to do that? What’s wrong with current methods for cancer diagnosis?
The current gold standard is to take a biopsy whenever a lump is deemed potentially non-benign by the clinician. This biopsy is then stained and analysed under the microscope by a pathologist. There are three major problems with this: first, the number of samples pathologists must assess is too high and they don’t have the luxury of spending a lot of time on individual samples. Secondly, at the initial stages, the differences between healthy and cancerous cells can be very subtle and therefore the assessment relies heavily on the pathologist’s experience. Besides, the number of cancer cells can be very low compared to the overwhelming number of healthy cells in the initial stages. So, it is extremely difficult for the human eye to spot those few cancer cells.
How is that different with the technology you are proposing?
Our technology addresses these three problems. We have developed an AI module which is able to identify single cells from large biopsy images and crop them out (more than a million individual cells are usually obtained from each biopsy sample). Subsequently, each cell is analysed individually. This allows our system to detect anomalies even if present in very few cells. These individual cells are then analysed by a second AI module which has been trained to identify and quantify patterns which are typically found in cancers. The advantage is that AI models can be trained on huge datasets and therefore, in a limited sense, acquire the experience that pathologists would usually take years to acquire. Finally, the system can give a grading for each sample (together with annotations) and enable the pathologist to allocate his time more productively to each sample.
How does AI determine whether a cell is cancerous or healthy?
Different compartments inside cells tend to have very specific and tightly controlled pH values (a measure of acidity). In several cancers, it has been observed that there is improper maintenance of pH in several intracellular compartments. Our technology builds on this knowledge to learn the pH patterns (pH fingerprint) which are found in healthy cells as well as those found at different stages of cancerous ones. It can subsequently compare the fingerprint it obtains from patients’ cells with what it had previously learnt and determine a probability of the patients’ cells being cancerous, along with the stage of the disease.
“The advantage is that AI models can be trained on huge datasets and therefore, in a limited sense, acquire the experience that pathologists would usually take years to acquire.”
Is this a totally new concept from the research done so far?
It would be both incorrect and unfair to claim that it is a completely new concept. That is rarely the case with scientific breakthroughs, especially in hot areas like cancer diagnosis. There are always a couple of teams from around the world collaborating with or racing against one another using their own strategies. Part of a scientist’s job is to keep track of what others in the field are doing in order to avoid redundancy as well as fine-tune one’s own approach according to findings from other labs. For more than a decade now, different approaches involving AI have been explored to improve cancer diagnosis. For example, the group I did my PhD with specialise in developing AI modules to identify patterns in the DNA in order to determine whether a cell is cancerous. Rigorous competition, collaboration, peer-review and validation ensures that a few extremely good configurations (usually integrating research from several teams), offering the best mix of accuracy and additional benefits, will eventually emerge and get adopted as standard practice in hospitals.
So what exactly is different in your project?
What we did differently in this project was to leverage on the knowledge that pH maintenance is generally disrupted in cancer cells, and that has worked out very well. In addition to a high degree of accuracy, the added advantages of our system include the ability to perform diagnosis without killing the cells, thereby enabling additional analysis on the sample. It also allows for the detection of a very low number of cancer cells, while being relatively inexpensive and fast.
How did it come about? I mean how did you move away from traditional research to this new type?
For about a decade now, an interesting change has been happening in the way academic research is conducted at leading universities and institutions around the world. Multidisciplinary and quantitative biomedical research is being heavily invested in and the returns have also been unprecedented. It is becoming clear that several biomedical problems require a multidisciplinary approach and I believe that this trend is only going to intensify going forward.
And how did you find yourself in the middle of all this?
I was very fortunate to be at the right place at the right time. While enrolled for a degree in biomedical sciences at the National University of Singapore (NUS), I had also taken several courses in physics, mathematics and computing out of personal interest. That subsequently allowed me to demonstrate a multidisciplinary approach for my final year research project. Fortunately, at the same time, an institute within NUS, the Mechanobiology Institute (MBI), was considering candidates for a multidisciplinary PhD programme. I was accepted for the programme and that is how I started to specialise in crafting a computer vision and machine learning solutions for understanding and solving biomedical problems. After completing my PhD, I joined a team that focuses on developing medical devices at the Department of Biomedical Engineering, NUS. It is this complementary fit which eventually resulted in this project.
“We have made tremendous progress in the last few decades, to the point that several cancers are now completely curable if detected in time.”
How long does it take to complete a project like this?
While the project, in its current form, was formally initiated in 2019, I have been developing these tools for many years now. For example, during my PhD research, I had developed a computer vision algorithm to identify and track individual motile cells in crowded populations. I had also created a module to extract them from large images and analyse them individually. At the time, those tools were devised to study fibroid diseases but have now been adapted for this project. So, it is generally difficult to give an exact duration for such projects. They are built on years and decades of research findings by our team as well as other groups from around the world. Whenever a breakthrough is achieved, it is important to recognise that it has the contribution of not only the team which achieved it, but also numerous past and present scientists from around the world.
I am also curious to know how you made your way from Grande Retraite and from modest backgrounds to The National University of Singapore?
After my CPE exams, I was accepted at the Collège du Saint Esprit for my secondary education. During that period, I was blessed with several incredible teachers, both within the school and outside, who nurtured my curiosity and intellectual interests. At the same time, my parents, relatives and close ones ensured that I had the right environment to give my best and excel in my studies. Upon the successful completion of my Higher School Certificate, I applied for several undergraduate programmes which I was interested in pursuing. After considering all the positive responses as well as scholarship/financial aid options, the NUS turned out to be the optimal choice for me. Thanks to the generous scholarship and financial aid available at the NUS, I was able to complete my studies and eventually pay back my study loan.
What is the next step from here?
Now that we have done the experimental validation and it has been peer-reviewed, we have started its clinical validation in collaboration with our clinical partners. We are currently optimising the system and exploring the feasibility of using this technology for detecting cancer cells from patients’ urine samples. The preliminary results look promising, so I am quite optimistic.
When, according to you, will we start making a dent in the fight against cancer?
Cancer is arguably among the most complex diseases and winning against it is one of the holy grails of medical research. We have made tremendous progress in the last few decades, to the point that several cancers are now completely curable if detected in time. So, while the fight is far from over and I am not able to give a timeline, we are already making an impact and it is only going to grow as we move forward.
What is the hope for cancer patients let’s say a decade from now?
The fight against cancer is on three main fronts right now, namely, the identification of susceptibility markers, timely diagnosis and effective treatment. Judging from current trends, the next decade is going to witness exceptional advances on each front. The dramatic drop in the cost of genetic sequencing has accelerated the pace at which we are identifying genetic markers for various diseases, including cancers. At the same time, our increased understanding of cancer and advances in engineering technologies is resulting in better diagnostic tools which can detect the disease at the very onset. We are also spotting encouraging returns on our investments in new fields like personalised medicine and gene therapy. All of these are being boosted by our ever-increasing computational power. So, a decade from now, I imagine more and more people being able to obtain their personal genetic profile along with their risks to certain diseases even before the onset. In cases where tumours develop without an underlying predisposition, we would still be able to detect them at earlier stages and provide customised and more effective therapies.
For you personally, do you have any plans of bringing your expertise back to Mauritius one day?
Irrespective of where I am, home is always Mauritius. I certainly want to contribute to Mauritius in whatever way and form possible. While I plan to return at some point, gaining as much global experience as possible is my current focus. Having said that, I believe that there are many ways to contribute towards the advancement of science in Mauritius and I am continuously exploring those.
Publicité
Les plus récents
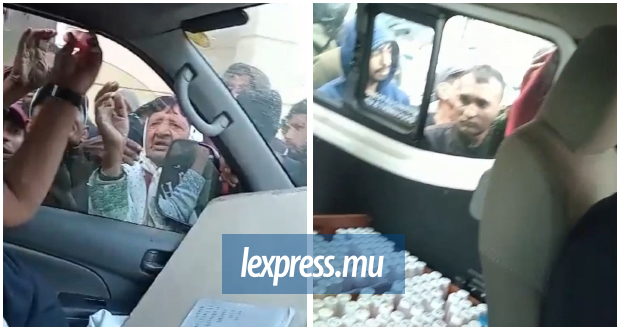
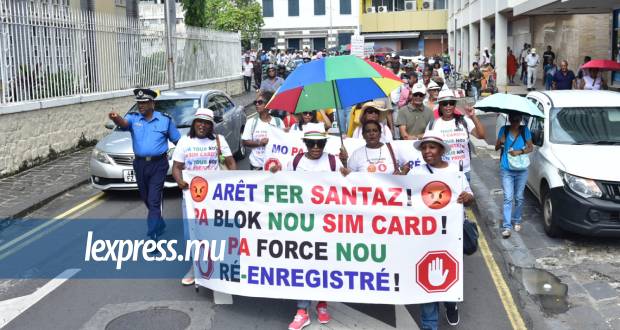
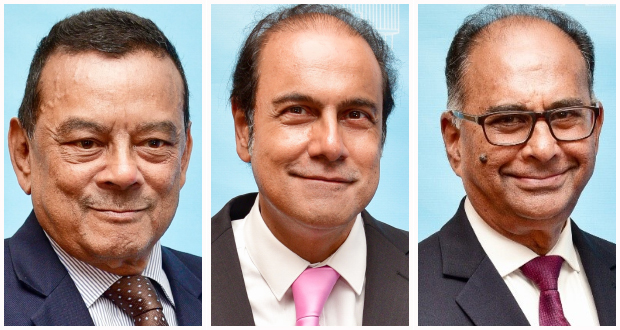
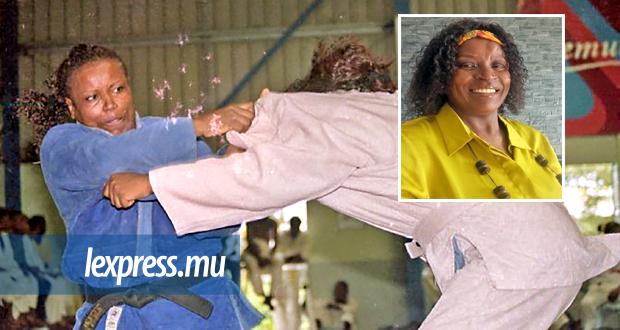
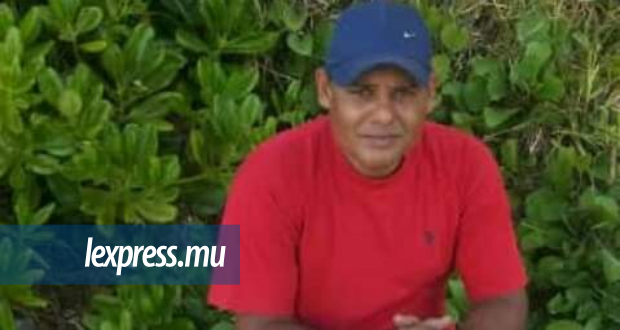